-
Ivana to give a talk at Duke Math Bio Seminar (Oct 2020)
-
Our paper is published in PNAS (Aug 2020)
-
Our paper is published in Nature Cancer (June 2020)
-
Ivana to give a Dartmouth Math Colloquium (May 2020)
-
Ivana to give a talk at Duke Math Bio Seminar (Oct 2020)
-
Our paper is published in PNAS (Aug 2020)
-
Our paper is published in Nature Cancer (June 2020)
-
Ivana to give a Dartmouth Math Colloquium (May 2020)
-
Ivana to give a talk at Duke Math Bio Seminar (Oct 2020)
-
Our paper is published in PNAS (Aug 2020)
-
Our paper is published in Nature Cancer (June 2020)
-
Ivana to give a Dartmouth Math Colloquium (May 2020)
Stochastic model of colorectal cancer initiation
We developed a stochastic mathematical model of colorectal cancer initiation
(PNAS 2020) through inactivation of two tumor suppressor genes and activation of one oncogene, accounting for the well-known path to colorectal cancer through loss of tumor suppressors APC and TP53 and gain of the KRAS oncogene. The model includes a complex network of 50 premalignant genotypes and 270 distinct paths on the way to colorectal cancer. Colonic crypts that have accumulated driver alterations undergo fission, and increase in number through a stochastic birth process. We find that the reported lifetime risk of colorectal cancer can be recovered using our mathematical model of colorectal cancer initiation together with experimentally measured mutation rates in colorectal tissues and proliferation rates of premalignant lesions. We demonstrate that the order of driver events in colorectal cancer is determined primarily by the fitness effects that they provide, rather than their mutation rates.

Quantifying genotype to phenotype map in CLL
We performed an integrative analysis of genetic data, clinical information and growth dynamics of chronic lymphocytic leukemia (CLL) (Nature 2019), including quantification of the effect of cancer mutations on CLL growth rates, improving our understanding of the relationship between genotypes and phenotypes in cancer. We derived an efficient Gibbs sampling algorithm that classifies the growth of CLL into categories corresponding to bounded (logistic-like) growth, or unbounded (exponential-like) growth, and found that each growth pattern was associated with marked differences in genetic composition, the pace of disease progression and the extent of clonal evolution. Finally, we inferred the distribution of growth rates of individual subclones and the differences to their parents using an MCMC-based method that samples an ensemble of likely phylogenetic trees for each patient, quantifying selective growth advantage of CLL driver mutations in vivo.
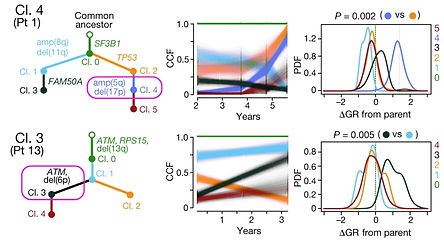
Evolutionary dynamics of resistance to cancer therapy
Using a multi-type branching process model for the accumulation of resistance mutations in growing tumors, we showed that radiographically detectable tumors are expected to harbor multiple mutations conferring resistance to any single targeted therapy prior to the start of treatment (PNAS 2014). Using used droplet-microfluidic technology and growth kinetic analyses, we demonstrated the presence of (often multiple) therapy-resistant subclones in CLL and estimated resistant subclone size before treatment initiation (Nat Commun 2016), confirming our theoretical findings.
We also evaluated the efficacy of combination targeted therapies (eLife 2013), and showed that dual therapy results in long-term disease control for most patients, if there are no single mutations that cause cross-resistance to both drugs; in patients with large disease burden, triple therapy is needed. We also find that simultaneous therapy with two drugs is much more effective than sequential therapy. Our results provide realistic expectations for the efficacy of new drug combinations and inform the design of trials for new cancer therapeutics.
​
​
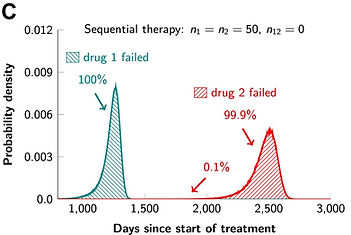
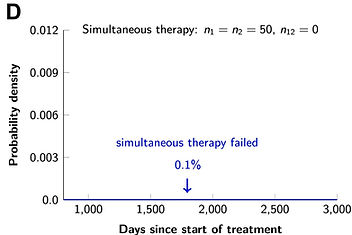